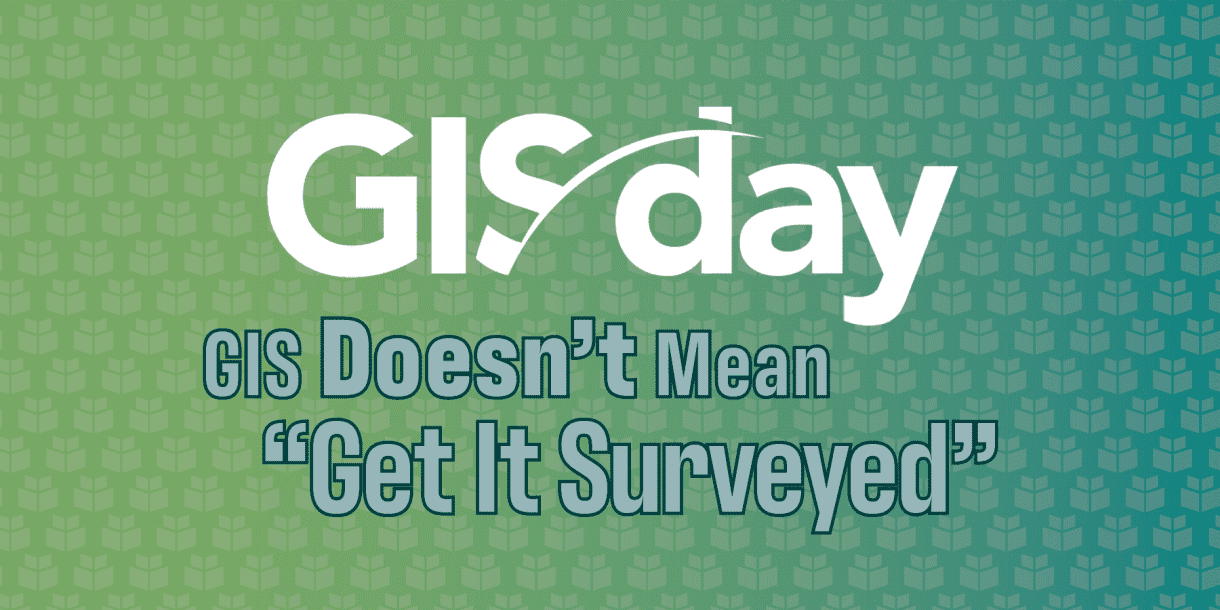
Introduction to GIS
Within the realm of geographic information systems (GIS), there are two major processes: data collection and data management. Data collection, the field portion of the work, involves using remote sensing equipment like the Global Positioning System (GPS), unmanned aerial systems (UAS), aerial and mobile LiDAR, robotics, and laser scanning to measure and record data like the size, position, and condition of infrastructure. Data management, the desktop portion of the work and the portion typically referred to as GIS involves organizing the data into a database that can be queried and rendered visually in tables, graphs, and, of course, maps.
While it would seem logical for data collection to precede data management we have to have data in order to organize it there are in fact significant benefits to approaching GIS from the data management side first. By asking What do I need to know?or What do I want to be able to do?we not only establish what fields and functions our database must contain, but also set ourselves up to leverage the tremendous power of the rapidly changing technology in the data collection arena.
Data Management
In order to answer these questions, it helps to understand what GIS can do, and what it cannot do. There is a negative perception of GIS in the surveying world, and it is summed up by the adage GIS stands for Get It Surveyed.Conventional surveyors often regard GIS as inaccurate and therefore a waste of resources why bother using GIS at all if we will have to go back and survey anyway?
But since GIS is, at its core, simply a vehicle for capturing and displaying large quantities of data, any inaccuracies in its output can be traced back to its input. If the original data is flawed or missing, it will be reflected in the GIS. GIS does not create inaccuracies it merely exposes them.
The reason for poor-quality or absent data is simple: historically, thorough and reliable data acquisition was too costly. High-quality equipment was expensive and the data collection process was labor-intensive. Securing the best equipment was no guarantee of success, however. For instance, there are measuring devices that advertise the ability to determine the location of a point in space at sub-foot accuracy, and in perfect conditions, sub-foot horizontal accuracy can be achieved. But the probability of working consistently in that environment is low, and in sub-optimal conditions, these devices may be off by many feet. And because each measurement is independent, achieving perfect conditions for one measurement does not guarantee them for the next. As a result, the recorded data represents varying degrees of inaccuracy, and the presence and degree of inaccuracy may not be readily apparent from the data itself.
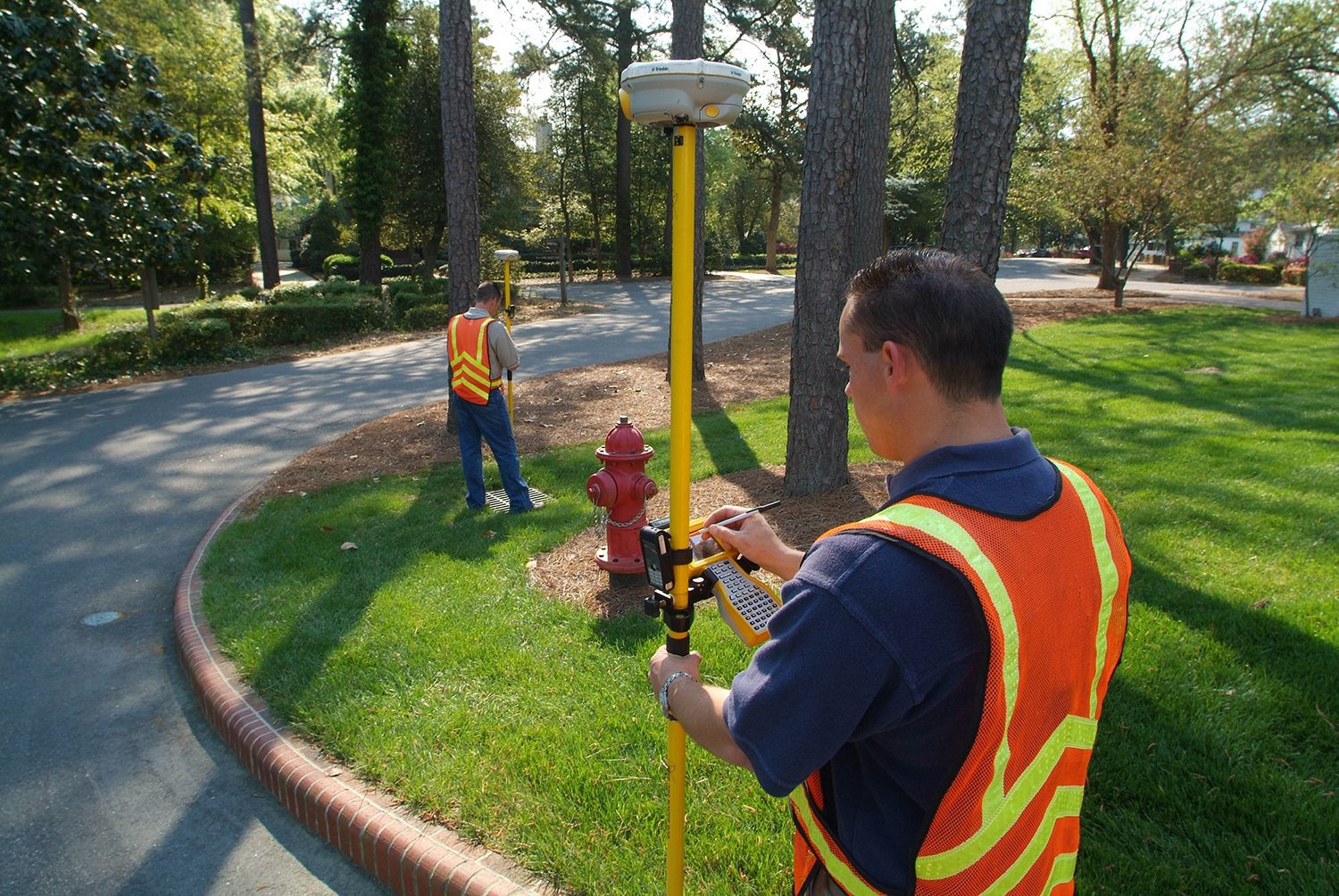
One critical way of detecting and combating these inaccuracies is recording and understanding metadata. Metadata is data that tells us something about other data. For example, a photograph is a collection of visual data, and a photo’s metadata might indicate what camera was used to take the photo or the speed of the shutter. GIS metadata can record information about the accuracy of a measurement using a metric called dilution of precision (DOP), which is the relative strength of the satellite configuration used to obtain a GPS reading. High dilution of precision indicates a high degree of uncertainty, or low accuracy; low dilution of precision indicates a low degree of uncertainty, or high accuracy.
We can record DOP for horizontal measurements, vertical measurements, or both. With older equipment, the GPS surveyor was responsible for understanding DOP and its impact on data accuracy. Today, many GPS units translate DOP metadata into an accuracy reading that tells us how far off a point’s recorded location is from its actual location in sub-foot or foot increments.
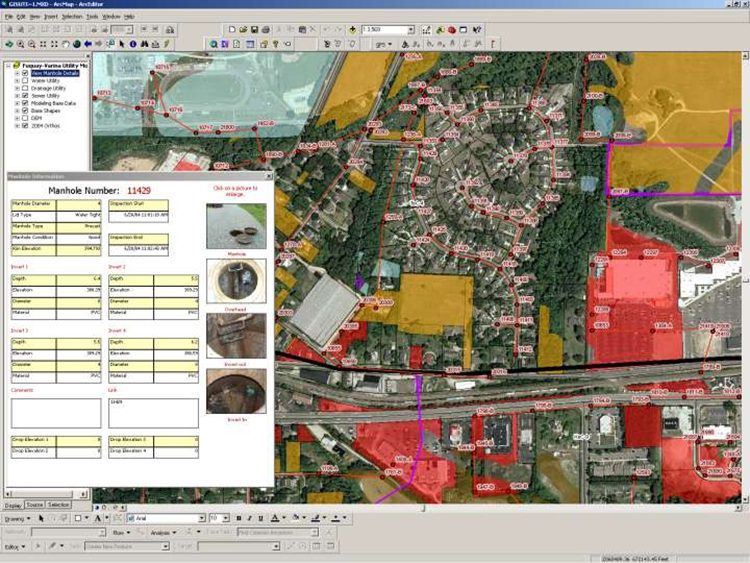
If this metadata is recorded and entered into a GIS database, we can review and evaluate the accuracy of the data for each asset, rather than designating the entire database goodor badwhen varying degrees of accuracy exist. We can also set thresholds for data accuracy based on how we wish to use the data in a specific instance: an asset management plan requires a moderate degree of horizontal accuracy but will be relatively unconcerned with vertical accuracy, whereas a hydraulic model absolutely depends on vertical accuracy. Any data that does not meet the threshold for accuracy for a particular application can be ignored.
With this in mind, the next step in using GIS after deciding what we want the data to accomplish is performing an audit of any data that already exists. A good audit not only determines what data is available, but also assesses the quality of that data based on the metadata. Areas where data is missing, and areas where metadata is missing or incomplete, are areas that will benefit from a fresh round of field verification or data collection, if needed, using all of the improved and new data collection tools at the geomatics professional’s disposal.
Data Collection
The technological advancements that have made digital cameras, mobile phones, and streaming media possible have had a profound effect on modern geospatial data collection. Data collection in the past versus data collection today is literally night and day: in the past, the number and location of satellites needed to perform GPS surveying meant that some field work had to take place in the middle of the night; today, we would have to be in dense canopy not to be able to get enough sufficiently strong satellite signals. Then, we had to set up our own base station; now, we can hop out of a car nearly anywhere and start surveying immediately. Counties that used to be flown for orthophotography shot in black-and-white at a couple feet per pixel are now shot in color at high resolution.
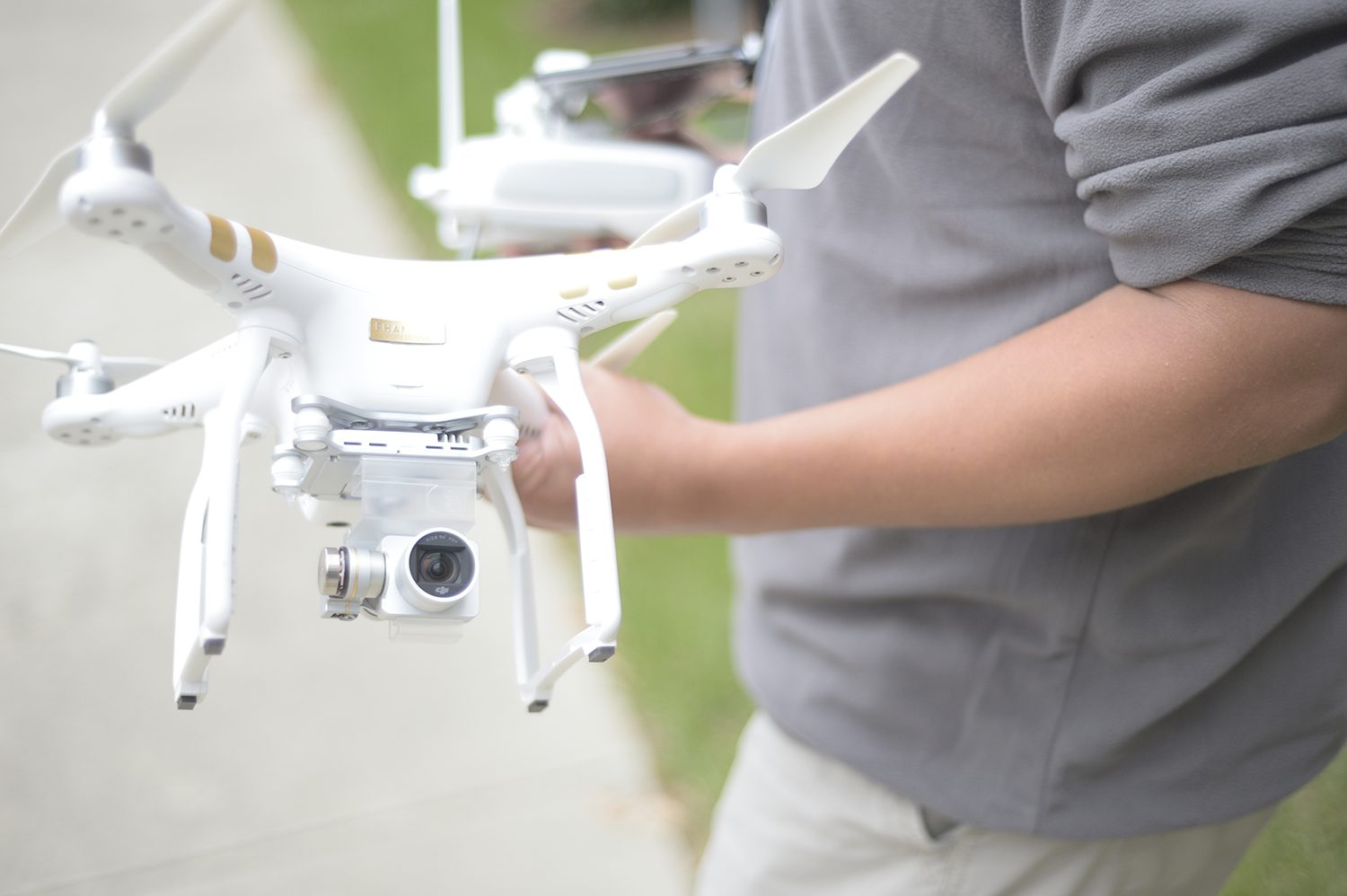
The miniaturization of data collection equipment is a key part of the changing GIS landscape. The camera that can be mounted on a UAS is as powerful as the camera mounted in a plane for orthophotography. This means that entities that used to have to rely solely on county- or state-wide orthophotography for GIS data can now isolate areas of change and use UAS to record, patch together, and orthorectify new data for their databases. The combination of old and new technologies enables more data to be collected more quickly, and at a lower cost.
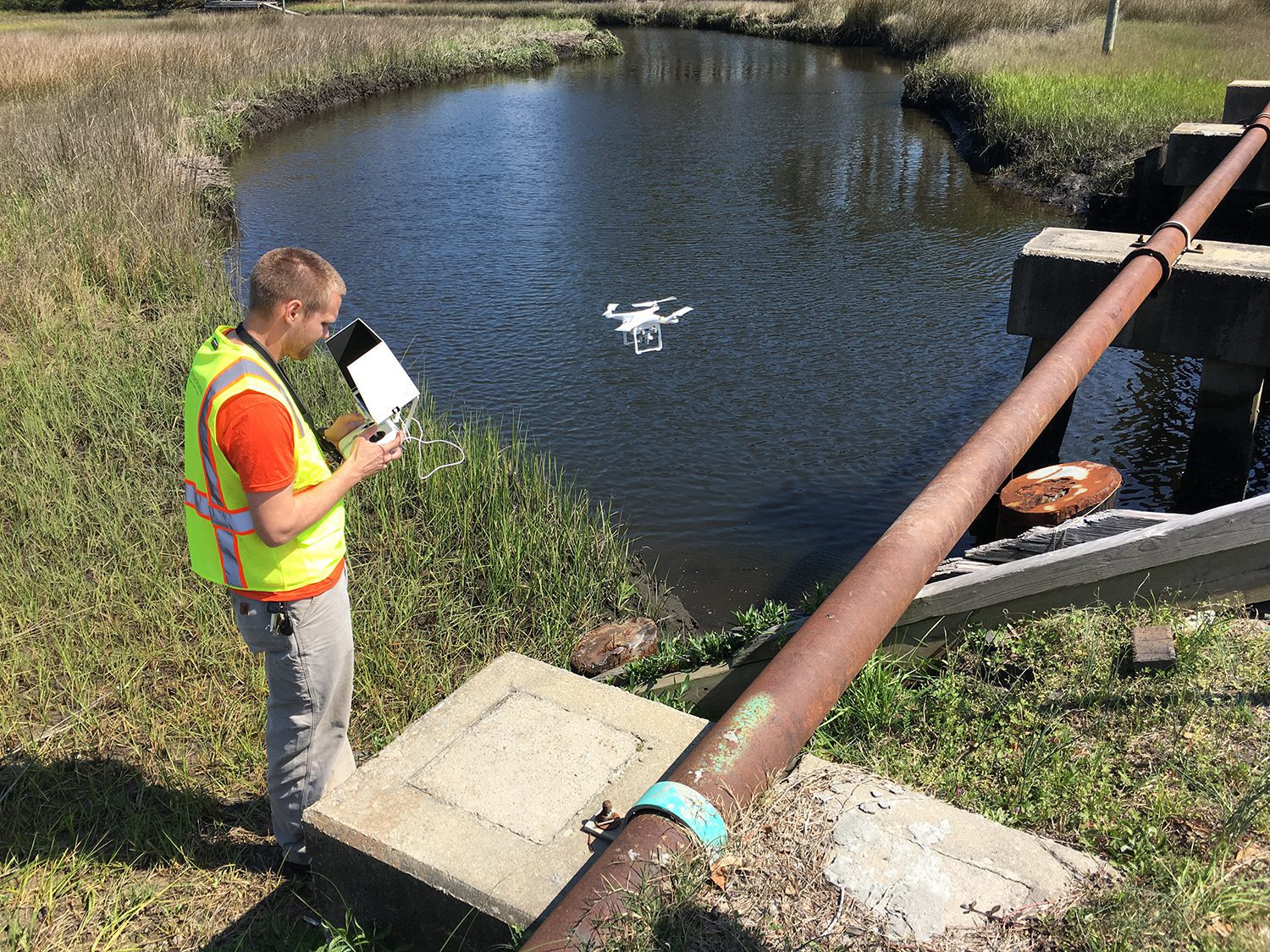
UAS can also be deployed to inspect difficult-to-reach places like water towers, aerial sewers, and the inside of large tanks; laser scanners, which capture millions of data points in three-dimensional space, are similarly useful in confined spaces like pipes and building interiors. Both technologies allow us to collect data that was difficult or impossible to obtain previously. They also take humans out of harm’s way and place them squarely in more safe environments.
All of these advancements in data collection drive improvements in data management, which in turn demand further refinements in data collection. As these two processes propel each other forward, the challenge for the GIS user and the layperson is understanding and manipulating the overwhelming volume of data available how do we transform that much data into information?
GIS
By employing sound data management principles like using metadata, and by taking advantage of rapidly improving data collection equipment and techniques, local and state governments and private interests can build a solid foundation of GIS data. With reliable datasets in place, we can start empowering them to act together and to inform the decision-making process.
Suppose we have a sewer system, and we know the service life of a specific pipe material is 60 years. So we query the database to find out how many linear feet of this pipe material are in our system, and of those, how many are approaching 60 years old. With this data, we might decide to CCTV those sections of pipe to determine which need to be replaced, and how soon.
During our CCTV inspection, we might discover that some sections of pipe are the same age, but display different degrees of deterioration. What might be the cause? We could review the database to determine what type of soil the pipes are buried in, or whether the pipes are located under pavement that takes a heavy load.
If we have connected our utility database to a work order system, we can look up how and how often the different sections of pipe are maintained. We can even see who inspected the assets, what issues they recorded, and what fixes, if any, were made. We are able to benchmark the performance of maintenance crews at the same time we are benchmarking system performance.
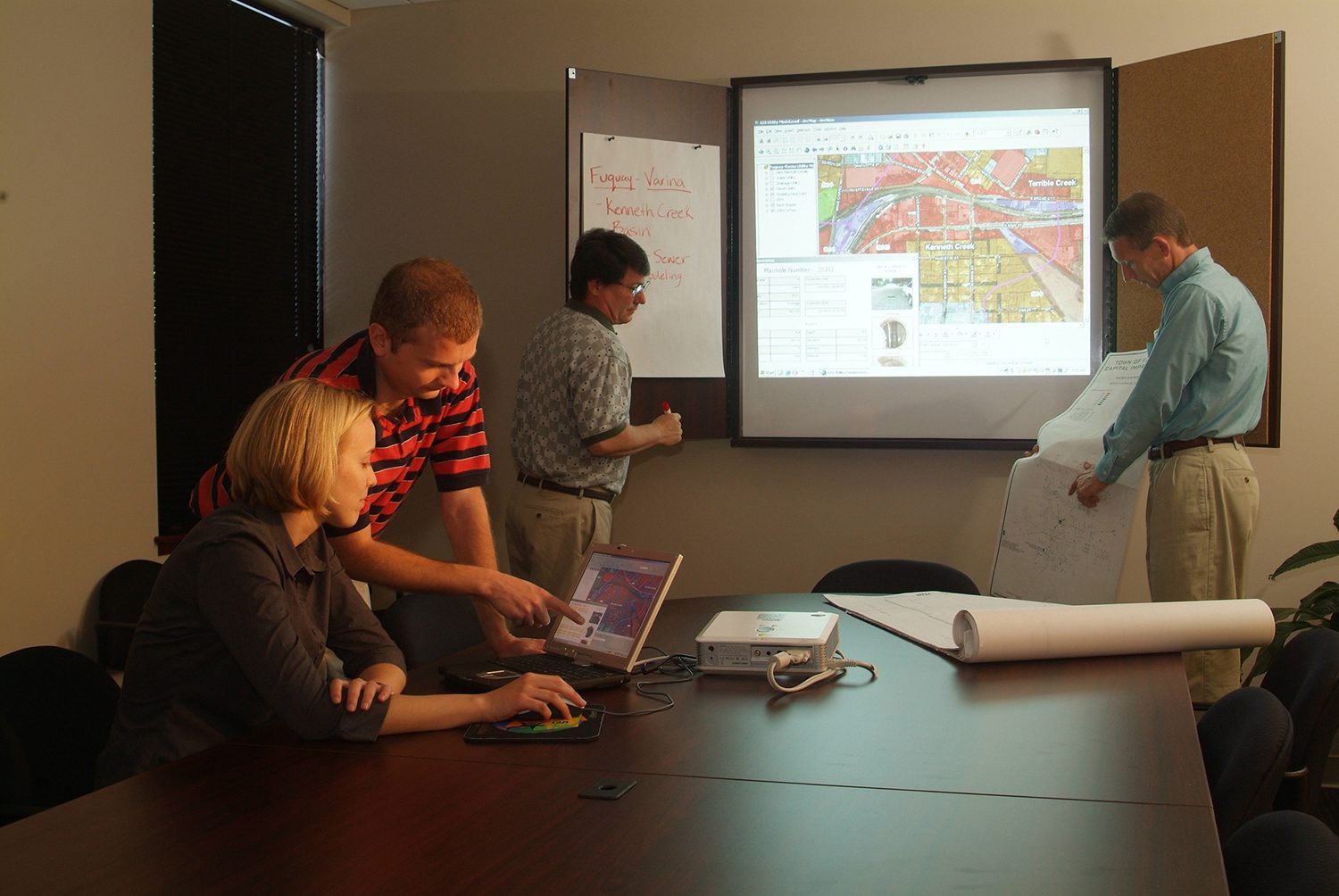
Through this process, we can recognize the signs of failure in one area of our system, predict failure in other areas, and move to proactively schedule and budget repairs and replacements. As other utility providers go through this process with their own systems, we can look to each other to increase our respective predictive abilities, particularly when evaluating the success or failure of different material and process changes in different contexts.
But to reach this interconnected and forward-looking place, we have to do a deep assessment of our GIS database. Is it functional? Is it accurate? Are we making good decisions based on our GIS? Are we even using GIS to make decisions? Once we figure out what gaps we have, we can bring to bear the full force of data collection and data management technology to fill those gaps.